Background
This Targeted IR&D project developed methods for automating removal of adhesives and difficult coatings from aviation components, without damaging the substrate. Aviation component remanufacturing is the targeted industry. Machine learning classifiers monitor and control the process controlling the system in a way to discontinue removal on completed areas, while completing areas where coatings remain. The system uses a robot outfitted with both a 3D camera for capturing the surface geometry and a color camera for classification. Tool paths, and then robot programs, are automatically generated, planned, and executed. A force-controlled end effector modulates the removal process, as required, over individual way points. The system repeats all processing segments until the designated surface area is fully processed. Fully completed waypoint segments are removed from subsequent passes to reduce overall processing time.
Approach
The proposed effort focused on developing a three-tiered classifier for coarse, medium, and fine abrasive media operations. Secondary proposed efforts sought to investigate alternate media, alternate processing geometry, and tool path generation strategies. Unexpectedly, the alternate media, combined with angled contact and interleaved and cross-hatched tool paths, provided excellent adhesive removal with minimal risk of substrate damage, using only the coarse process. Classifier training tools were also developed to simplify system setup for different coatings, adhesives, and substrates.
The nominal solution, employing a random orbital sander, was tested initially and discovered to be ineffective at removing the difficult coatings, even with very coarse media and substantial normal force applied. A scouring pad style media mounted on an angle grinder was tested at varying contact angles. This proved surprisingly effective at removing the adhesive, but also carried a large risk of damaging the substrate. Noted during the evaluation was the contact patch for an angled approach is smaller and its location is sensitive to accurate normal estimation. SwRI therefore implemented a unique surface normal and trajectory filtering methods ensuring processing would be applied only where desired.
Accomplishments
The effort demonstrated end-to-end processing of large surfaces of delicate substrates with hard coatings. In addition, the following technologies were developed and demonstrated:
Tool for quickly training image classifiers for material removal processes.
Reliable normal estimation, especially near edges, of scanned surfaces.
Advanced tool path generation for interleaved, cross-hatched tool paths.
Intelligent selection of media and processes for material removal.
Automatic removal of raster strokes from a path plan once a degree of completion is realized using sensor-based process monitoring
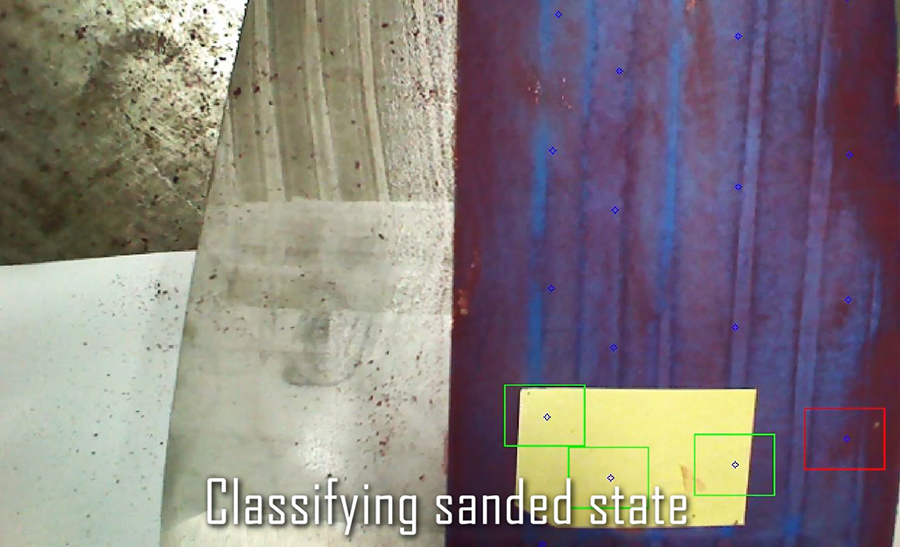
Figure 1: An automated system removes a coating as part of the development of a composite component reprocessing system (left). An abrasive media disk contacts the surface at an angle, concentrating applied force at the affected area. An image classifier modulates the applied force at each waypoint based on the assessed state, red continue applying force, green reduce force to zero.