Background
The Human Performance Initiative at SwRI has developed a markerless biomechanics system to capture human motion data in any environment with accuracy that rivals laboratory-based motion capture systems. This technology applies to the fields of peak human performance, medical diagnostics, and veterinary/zoological sciences. While the currently deployed system processes video data across multiple cameras, the neural network backbone is only looking at single frames and making predictions based on that small datapoint. By using emerging neural network architectures and training pipelines, we can incorporate temporal aspects of human motion into the markerless motion capture pipeline, creating a network that understands the context of the human body in motion.
Approach
The temporal aspect of this neural network design required restructuring the underlying dataset used to train the network. We incorporated three publicly available training datasets and two internally collected datasets for gait and functional movements, reorganizing the data to sample multiple consecutive frames.
Significant network restructuring was necessary to handle the multi-frame approach for this project. Functionally, every additional frame that is passed to the network adds significant training time and increases the size of the network. To handle this issue, we first implemented the PyTorch Lightning library, allowing faster training across multiple GPUs.
The goal of the project was to build a network that simultaneously made temporal and spatial predictions. To accomplish this, we used an additional convolutional dimension in the network that allows it to communicate information between frame predictions. This also makes the network easily scalable to different sequence lengths as necessary. Due to the additional network size required for this approach, and the inherent training time increases for the increased input data, we implemented these network changes in a streamlined version of the original architecture known as Lite-HRNet.
Accomplishments
We built and trained spatial-temporal networks on sequence lengths of one, two, four, and eight frames. The single-frame implementation of the network will serve as the baseline against which to compare the temporal improvements. Figure 1 shows the root mean squared errors (RMSE) of all four networks across all degrees of freedom for the countermovement jump motions within the testing dataset.
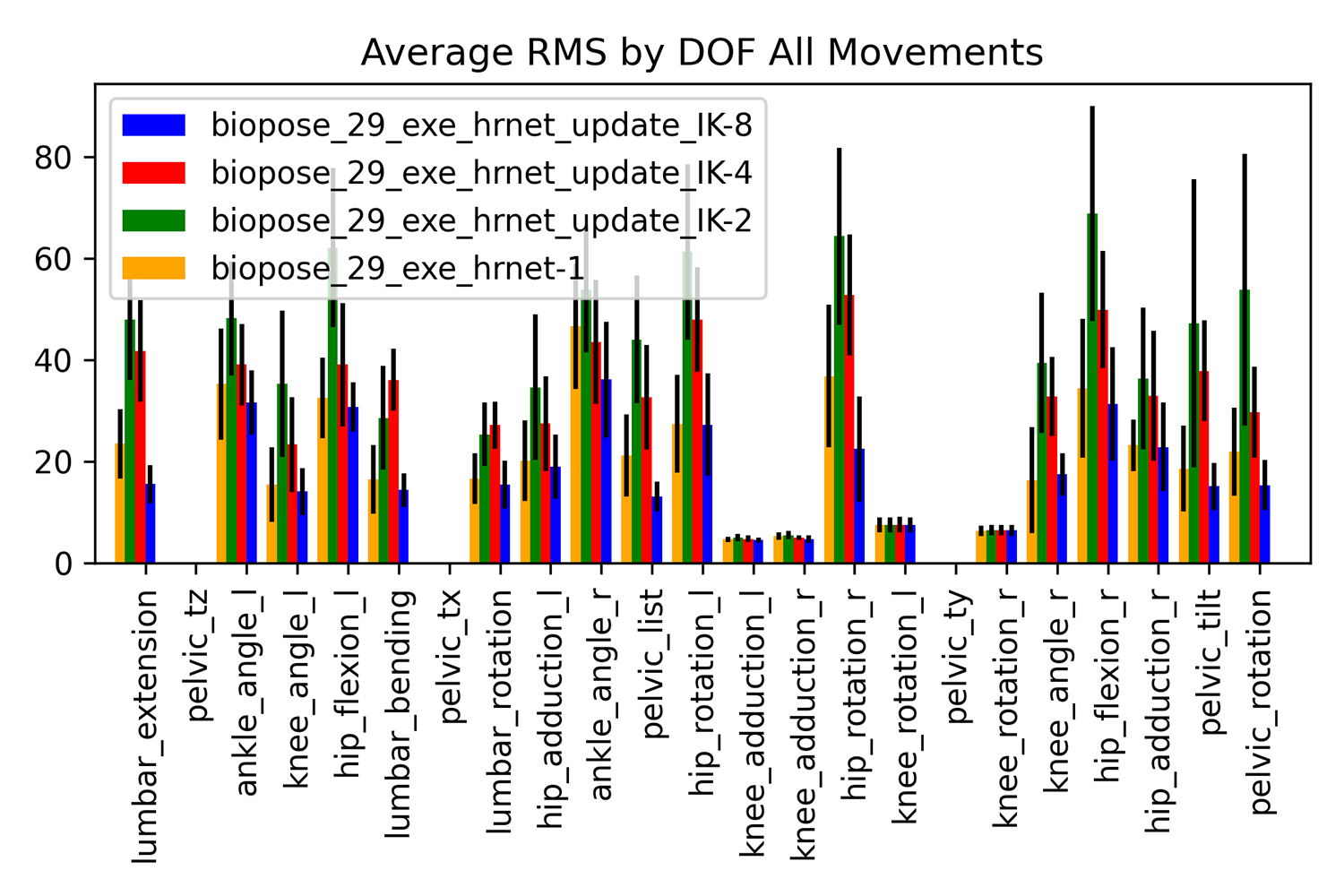
Figure 1: RMSE for all networks and degrees of freedom.
This shows that initially adding only a small number of sequential frames of data (two) had a negative effect on the accuracy of the network, but as the sequence length increases, the errors reduce and a sequence length of eight frames results in better results than the base Lite-HRNet network, an 8% increase in accuracy.