Background
In a marketspace that generates approximately $800 billion annually, the textiles industry is still heavily reliant on manual labor. One main cause of this is that manipulating flexible materials is inherently difficult, and the process requires a feedback system that can observe and correct the fabric during high-paced sewing and cutting operations. Current manual operations can adjust the fabric, but every adjustment creates a quality risk of fabric misalignment, stitch defects, etc. By automatically applying these corrections via a feedback system operating at or above the manual rate, the fabric can be positioned where needed throughout the entire process, enabling use of robotics in textile sewing.
Approach
The objective of this research was to develop a capability for calculating seam pattern trajectories that are robotically printed onto a fabric substrate in transparent ink that fluoresces under ultraviolet (UV) light. Once detected, the vision system computes the seam trajectory error as compared to the nominal. We developed an image processing pipeline that consisted of four steps: Gaussian blurring to reduce noise, binary thresholding to remove low-intensity pixels representing areas without ink, morphological closing to fill in gaps, and skeletonization to reduce the detected print areas to single pixel-wide lines in the image. We used fiducial tags printed with the seam pattern to correspond the measured to nominal seam trajectory. Once the tags were detected in an image of the fabric, the corners of the tags and their correspondences in the seam pattern were used in a perspective-n-point (PnP) optimization to calculate the transformation from the camera to the detected pattern. This transformation (along with the camera’s intrinsic parameters) was used to project the model of the seam (i.e., the pattern) onto the detected seam in the camera image (Figure 1). The difference between the seam model and the detected seam was calculated as the Euclidean distance between each point in the seam model and its nearest neighbor in the detected seam.
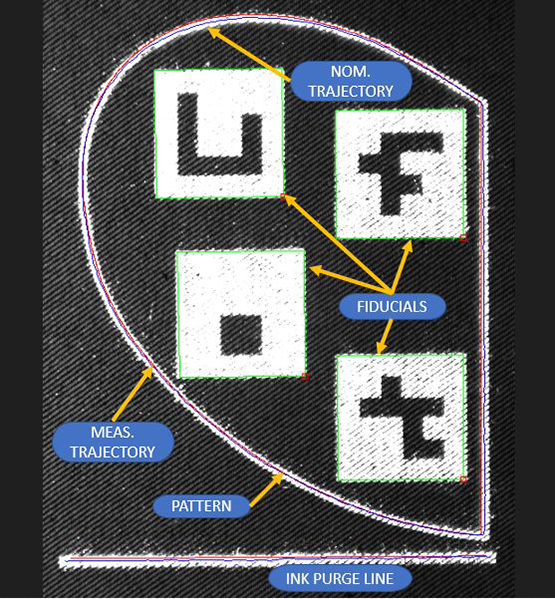
Figure 1: Pocket pattern on denim (white), in jet printed seam (red), measured trajectory (blue), nominal trajectory (green), fiducial identification, and purge line used to stabilize ink system.
Accomplishments
Several key results were achieved from this project. One main discovery was the effect of fabric types on print quality and error estimates. Since materials like denim use thick yarn, the ink deposited on the surface mimics a dotted line instead of a smooth line, as the ink is not able to penetrate the gaps created between yarns. In contrast, synthetic fabrics allow the ink to leach with much less resistance, resulting in blurred pattern lines. Due to the flexibility of the ink jet system, we were able to establish ink jet parameters compatible with the imaging system for natural and synthetic fabrics.
Figure 2: Video clip showing pattern tracking and average deviation.
To quantify the seam tracking error, we used a tolerance interval assessment. The analysis indicated that a project goal of a 1mm tracking error for knits and a 1.5mm error for denim was met on both white t-shirt and denim fabrics, respectively. For camouflage samples, we calculated seam tracking errors in excess of the goal which resulted from two factors: non-rigid warping of the pattern, such as partial fabric shear and wrinkling, and identifying less than two fiducials which are needed to correspond the measured to nominal pattern. Future work to modulate the exposure time and illumination is expected to improve tracking performance with camouflage fabrics.