Background
In recent projects, Southwest Research Institute® (SwRI®) has demonstrated the ability to remotely exploit automated vehicle sensors. Customers are interested in the vulnerability exploitation but are more focused on solutions to mitigate these threats. The proposed research is focused on detecting erroneous data in real-time on vehicle sensor networks. Cyber-attacks such as remote spoofing and lower tech issues such as being obstructed or damaged, is detected by using machine learning-based algorithms tailored for anomaly detection. The Intellectual Property developed on this project supports incorporation into customer vehicles (both military and commercial) as an independent system that can flag issues with sensors that would be difficult or impossible to detect from access to a single sensor output. Implementation of this technology improves security and increases safety in automated vehicles and Automated Driver Assistance Systems.
Approach
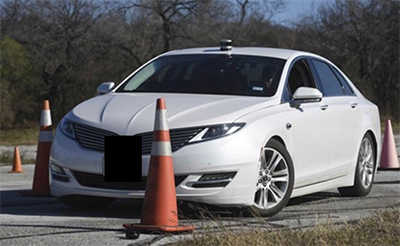
Figure 1: SwRI-Owned Vehicle Outfitted with LIDAR, GPS, wheel speed, and other sensors.
The objective of this research is to accurately detect erroneous and spoofed sensor data in autonomous vehicle sensor networks in real-time. This ability is critical to address customers’ concerns of cyber-security and information assurance in autonomous vehicles. The research focuses on velocity data extracted from LIDAR, GPS, and Wheel speed sensors in a SwRI-owned autonomous vehicle. The resulting system will read in raw sensor data in real-time, extract velocity information from LIDAR, pass velocity information from all the sensors into the detection algorithms, and flag anomalous data including identification of the problem sensor. Anomalies will be created from collected data and inserted into a live data feed to test the system. The vehicle used for the project is shown in Figure 1.
Accomplishments
The team collected sensor data and created data sets to use in developing and training the detection algorithms. The team is currently developing the detection algorithms and integrating with the test vehicle for real-time performance.