Background
Chromosome instability (CIN) and aneuploidy are classic hallmarks of cancer, caused by cells deviating from the normal cell cycle. In a tumor, CIN is variable from cell to cell and can result in many forms of aneuploidy, including changes in chromosome structure, number, and arrangement. Polyploidy is a form of aneuploidy that results in several nuclei within a single cell. Polyploidy has been found in numerous cancers and is related to therapeutic resistance. Currently, there is no effective method to identifying polyploid cells due to the high degree of similarity between them and surrounding cancer cells. Automating the identification of polyploid cells using Artificial Intelligence (AI) could aid drug development and research efforts targeting polyploidy.
Approach
A custom neural network was trained to identify polyploidy in tissue images. A detection algorithm produces a set of bounding boxes corresponding to each detected polyploid cell, after which duplicate/overlapping boxes are eliminated, and then feature vectors are sampled from an earlier layer of the neural network. These features are fed into a second convolutional neural network that predicts which pixels are part of that polyploid cell. Images of mouse liver tissue that contain polyploid cells were obtained from Dr. Daruka Mahadevan’s lab at the University of Texas Health Science Center of San Antonio (UTHSCSA). With assistance from hematopathologists at UTHSCSA, polyploid cells in 30 images were labeled as 4n-, 8n-, or cellular polyploidy, and used as input data to train the custom machine learning algorithm. The dataset was iterated over for 300 epochs to solve for the model parameters. The trained algorithm was tested on 25 new images from the same set of mouse liver data. The testing labels were corrected, and the training process was repeated with the 25 new images combined with the original 30 training images. The retrained algorithm was then tested on 32 new images.
Accomplishments
Through this internal research project, we developed a labeling tool that imports images of cells and offers several different labeling techniques and evaluation methods. The custom algorithm predicted polyploid cells with 94.4% accuracy and predicted sub-classes of polyploidy with 86.7% accuracy. This work demonstrates that machine learning can be used to perform instance-aware semantic segmentation to identify polyploid cells within tissue biopsy images. Our algorithm was trained and tested on healthy mouse liver cells because they are easily available and naturally contain unharmful polyploid cells. Future efforts will focus on implementing the algorithm on lymphoma biopsies and using the algorithm to assess how well a drug can prevent polyploidy when administered with chemotherapy. With further research, the use of AI polyploid identification in patient samples could allow clinicians to use polyploid severity as a predictive marker of therapeutic resistance and modify therapeutic regimen as necessary.
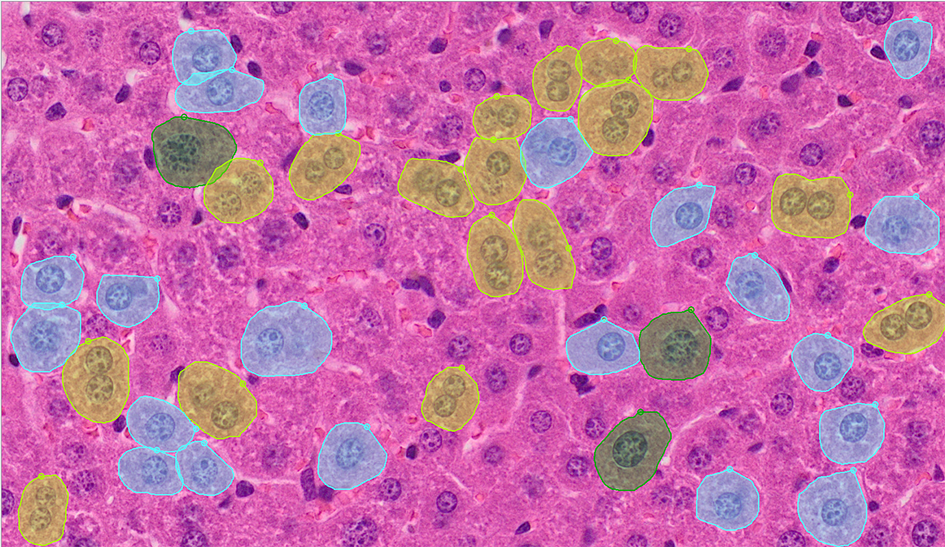
Figure 1: Three types of polyploid cells detected via an instance-aware semantic segmentation algorithm. The yellow labels indicate multinucleated/cellular polyploid cells; the blue labels indicate 4n polyploidy; and the green labels indicate 8n polyploidy.