Technology Today sat down with Dr. Steve Dellenback, vice president of SwRI’s Intelligent Systems Division and a leader in the automated vehicle field. We discussed this rapidly evolving industry and the next big barrier: How do we program a car’s decision-making system to weigh the ethical implications of its actions?
Technology Today: There’s a lot of automated vehicle hype out there. When do you think we will see automated vehicles on streets and highways?
Steve Dellenback: I think we’ll have automated driving systems in constrained areas in the next two to five years. These environments could include mining operations, farms, freight yards, or possibly places like retirement communities where there isn’t a lot of traffic. I think we’re probably 10 to 20 years out for full mixed-mode, with human-driven cars and trucks interacting with vehicles equipped with automated driving systems. If there were managed “technology lanes” set out on highways where only vehicles with certain technologies could drive — similar to toll lanes — I think we could deploy it a lot sooner.
TT: What keeps automated vehicles, or AVs, from being adopted more quickly?
SD: One reason is cost; the enabling technologies are not cheap. The average price of a vehicle in the U.S. is now about $34,000, but you’re looking at tens of thousands of dollars more for the hardware that enables automated driving operations. Another reason is perception. People sometimes don’t understand how capable the human eyeball and brain are. You look out at the road and you can easily identify hundreds of objects. The crossing over of information between your eye and your brain is truly astounding. Trying to recreate the same fidelity as the human eye for automation — frankly, we’re still not there. Now there’s a third aspect on the horizon: cyber security. Since its invention, the car has been a stand-alone device, unconnected to the outside world. The addition of communications integrated into the vehicle introduces another whole set of issues, including how do you prevent people from hacking into cars? But perhaps the most serious hurdle is the societal issue, psychological acceptance. Will people trust a vehicle equipped with an automated driving system with their life?
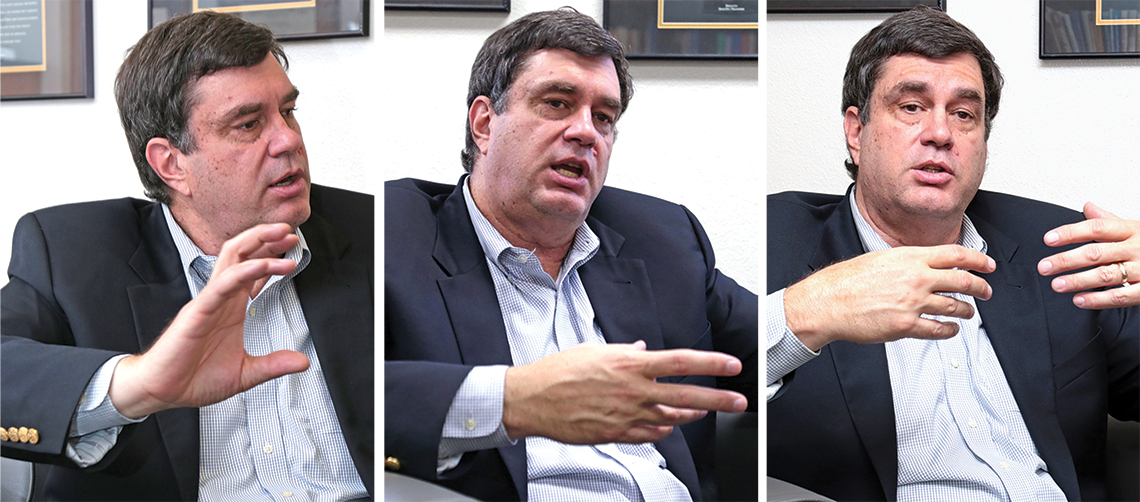
Dr. Dellenback has over 32 years of research and development experience in a variety of areas ranging from microprocessor assembly language, to large integrated factory floor automation, statewide integrated transportation systems, and automated vehicles. He discussed SwRI’s program in automated driving and how complicated the development process remains.
TT: How do you balance cost-effectiveness and performance?
SD: Perception-based behaviors are challenging. There’s a big tradeoff in the capability of sensors. You could buy a very expen-sive camera and have fantastic resolution at a long distance. But the typical commercial car can’t absorb adding thousand-dollar sensors, so manufacturers are looking at cameras in the $30 to $100 range. To make good driving decisions, you need to see about 80 meters in front of the car. But if you look at a traffic light at that range through a very low-end camera, you may only have two to four pixels of camera resolution from that light. How does the computer know it’s a traffic light and not just a light alongside the road? That’s the real issue: How do you get enough resolution to discriminate? That’s where the human eye has amazing resolution and where the human brain has the ability to understand context.
Cameras are just one kind of sensor. You’ve heard about LIDAR; that’s the spinning-light laser. LIDAR is very good for detecting large objects — a car, a house, or a tree. It identifies the edges of that object. But if you have LIDAR and you’re in tall grass, it’s going to think the grass is a solid object. While 65 percent of the roadways in this country are paved, that means 35 percent are not.
That’s why you have to start fusing technologies, such as adding radar. Higher-end cars have been adding advanced driver assistance systems, or ADAS, for a number of years. Adaptive cruise control uses sensors to measure the distance to the car in front. Some cars have little infrared sensors along the bumper. They’re good from two to eight feet, and that’s how you sense distance when you are auto-parking or when you’re pulling into your garage and it beeps when you are too close to the pillars next to the door. The trick is to fuse these different sensor technologies at as low level as possible.
And then there’s weather. It’s one thing when there are blue skies, but let’s consider how rain affects sensor performance. Then turn the rain into snow. And then let’s turn the lights out and be in total darkness. With a $100 camera in the dark with no streetlights, you can’t see anything. Sure, there are night-vision cameras, but those run in the thousands to tens of thousands of dollars. This whole sensor fusion concept is necessary and complex if you truly want automated vehicles to work in all operating modes, especially when you consider the need for sensor redundancy.
TT: But even with the right technology package onboard, that won’t necessarily solve all the problems.
SD: Right. Consider the “Trolley Problem.” This classic, 100-year-old ethics problem, where a trolley is coming down a hill out of control, and you’re standing next to a switch. You can choose for it to go left or right. On the left-hand track there are four people, and on the right-hand track there’s one person. The ethical question is, which way do you throw the switch? Most people quickly say they’d choose the right track, with just one person. But I forgot to tell you — that one person is your child or an important political figure, which further complicates the value question.
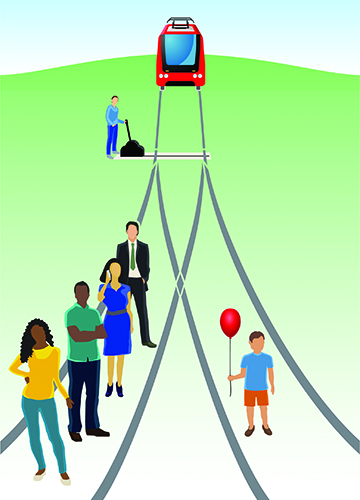
The Trolley Problem: An out-of-control trolley is racing downhill. Would you send it left or right?
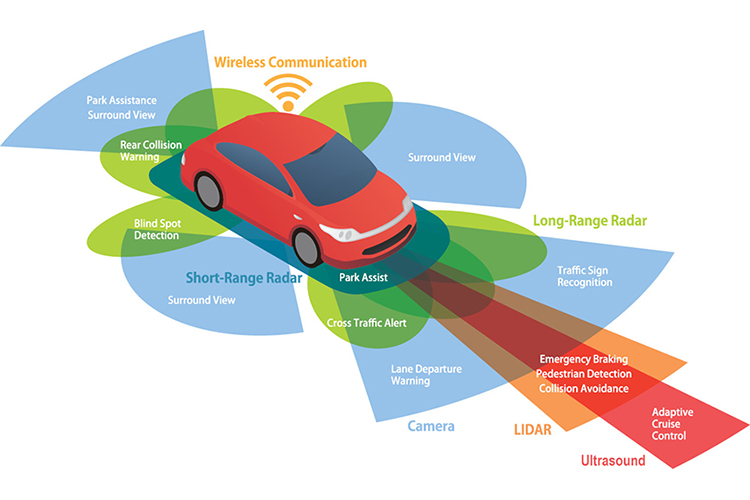
By 2050, the penetration of automated vehicles could reduce traffic fatalities in the U.S. by as much as 90%, saving almost 300,000 lives every decade and $190 billion a year in medical costs associated with accidents.*
T: How do you build ethics into an autonomous system?
SD: In my mind, ethics entails creating a “value proposition.” At some point, you have to start putting values on things — minor crashes, major crashes, what kinds of objects you can hit. You have to figure out what the system can do, and see us do, in our everyday driving that we didn’t really know we were doing. For instance, when you see an object in the road you make a decision: You might put two wheels off on the side of the road and go around it. The real question is whether to build that response into the program. That’s somewhat new ground in this industry, and I don’t think many people are really working on building in ethics. Ethics are relative to personal values, and personal values vary by geographic region, upbringing, a myriad of things. Do you want the vehicle to adapt to the consumer’s values, or do you impose the same values/ethics on all robots? We are still trying to figure out how to get them to fundamentally drive, to fundamentally prove themselves to be a safe and reliable product. That’s why I think we are still not just three years out, because of the ethical implications.
TT: Can an automated car be given a conscience?
SD: We all make value decisions related to driving. But driving styles and driving conditions vary. As humans, we sense that in certain conditions we should slow down, and that plays into how we make decisions. If you’re driving through a neighborhood and you know there’s a bunch of kids there, most people will drive a little slower. How do you build something like that into a vehicle? Human drivers are making decisions based on our environment and our values and our understanding of the consequences of pain. Robots don’t yet feel, or react to the concept of pain. And even if they did, what would their pain threshold be? Some people simply won’t slow down. They’ll say, “I’m going to drive full speed through this neighborhood because it’s my right to drive 30 miles an hour.” Someone else who is a parent or a grandparent might say, “I’m going to drive 20 miles an hour because that’s the safer thing to do.” At a different time of day, say 11 p.m., maybe it’s fine to go 30. But where does that point break? And then you throw in things like holidays. Let’s say it’s Halloween. That requires a change of driving style, because typically, children are smart enough not to run out in front of cars. But on Halloween, all bets are off. So there’s that whole aspect of changing styles based on environments.
TT: So the automated driving system must not only learn the rules of the road but also quantify the risks of complex scenarios and make financial and/or life-or-death decisions?
SD: AVs are going to have to decide if and when they should brake or swerve off the road. Let’s say there’s a moose in the road: You and I both know logically that if you hit a moose, you’re in a lot of trouble. The question is, should you veer off the road to miss it? If you veer one way you may enter the path of an oncoming car; if you veer the other way, you could end up in a ditch. Your software, your systems have to be smart enough to compare the risk of hitting a moose or swerving. If you’re on the Bonneville Salt Flats, it’s easy to veer off. But if you’re about to hit a moose, you could be in the Grand Tetons on a mountain road. If you veer off the side, you could plunge 1,000 feet.
Consider another scenario: You are in the middle of a crowded highway or on an icy road and a dog wanders onto the road. Braking or evasive maneuvers would be dangerous, and many of us know that in some situations you probably should stay the course. But what if it isn’t a dog? What if it’s a child crawling? Should we trust our computers to distinguish that? It looks like it has four legs; it could look like a dog to the computer, but with the human eye that’s a nonissue. So how are we going to get a computer to do that? Frankly, that’s beyond my programming skills.
TT: How do you get computers to learn how to drive when there are so many variables involved?
SD: We are using an advanced machine learning technique known as deep learning. You essentially teach patterns to the computer, and the computer eventually starts extrapolating those patterns. A simple example is, you teach a computer what a human walking toward you looks like, and then you teach the computer what a car looks like. So then, what if there is a person walking behind a car, where you only see part of the body? With deep learning, you don’t have to program into the computer what a body looks like when it’s behind a car. You’ve already taught it what a car and a body both look like, so the computer will infer that the human is behind a car. Computing power has finally gotten to the point that it can support real-time deep learning implementations. In past years, deep learning was very much an offline, post-processing activity. With the dynamic driving task, response times have to be in the milliseconds. When a car is driving 70 miles an hour, you can’t think about it for a half-second; you have to act very quickly. So that’s why deep learning is now coming into its own in this field. Actually, the Institute is applying deep learning to many different aspects of its research, in a number of divisions and a number of technology areas. Keep in mind, machine learning is not the end solution to every problem. It is just one tool that is popular right now. As technology continues to evolve, we believe deep learning will be part of a solution but not necessarily the end solution.
TT: How will deep learning affect the next phase of automated driving implementations?
SD: Deep learning is basically thinking. You’re trying to get the computer to draw conclusions. That’s scary, because if you talk to an auto company or a Tier 1 supplier, they tell you that they normally want about 300 million miles worth of data on a vehicle or a new system to declare it safe and ready for production. When we build these deep-learning systems in the laboratory development environment, we train them using a significant database of images so the computer can “learn” what components it is looking for in a scene. When these systems operate on a vehicle, they are analyzing sensor data to look for patterns they were previously taught to recognize. So, you put them in a car and they start learning, conceivably becoming better and better drivers. However, the question is, who is doing the teaching? Is the vehicle learning how you drive, which is probably fairly safe? Or how your teenage son drives? So how do you make sure that your system is learning right? We are trying to come up with a technique for validating these deep-learning networks. How do we know that it is doing the right thing, repeatedly?
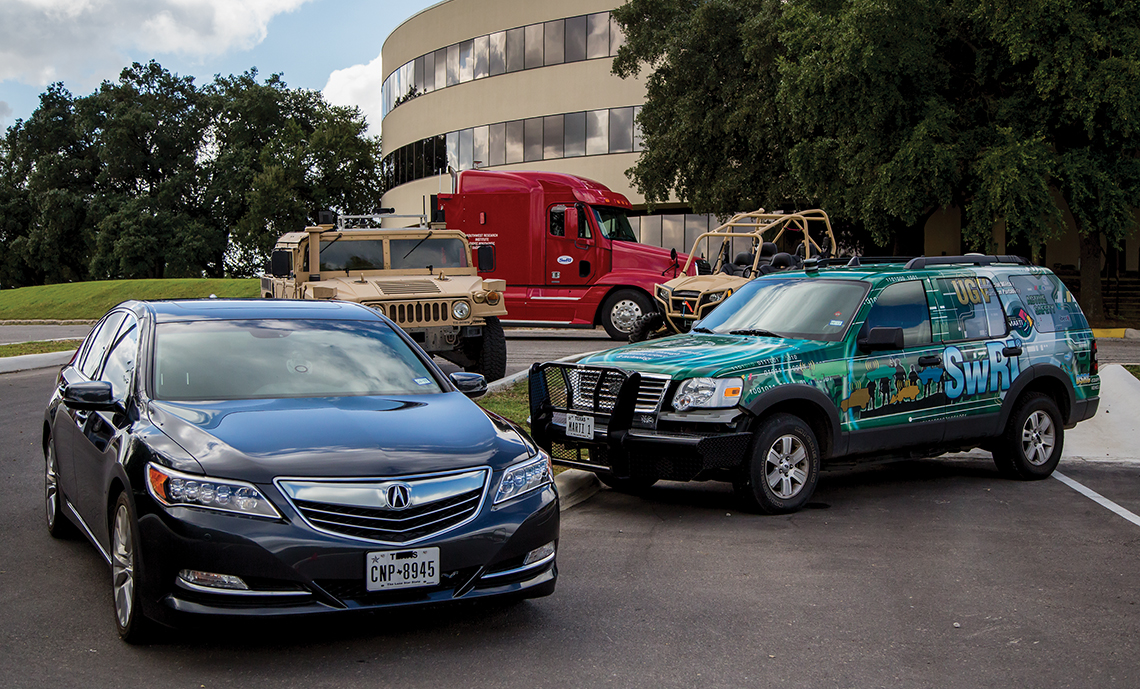
In 2016, SwRI’s automated vehicle program celebrated its 10-year anniversary. Pictured are five of the 30 vehicles in our unmanned systems research fleet. We develop low-cost, high-performance perception, localization, path planning, and control technologies for intelligent vehicles ranging from golf carts to SUVs to Class 8 tractor trailers.
TT: What do you think is the most important automated driving system goal?
SD: We talk about a crash-free society as the ultimate goal. If you look at traffic deaths, last year they rose by approximately 5,000 people. For the first time in many years, 40,000 Americans perished in auto accidents, and 93 percent of the unimpaired crashes were categorized as human error. But can you get to a crashless society? I think the idea that automated cars will never crash is unrealistic. Recent events in the news suggest that’s a very difficult thing to do. As an engineer, I think it’s a myth to say they will never crash. But I think we can get very close to crash-free using driverless technology. But we still have many hurdles.TT: Where are we today in implementing automated
driving systems?
SD: Lots of cars currently have some automated functions right now, but they are not fully automated. With automated driving systems, how long does it take to get your attention back on the wheel? How long does it take for your mind to get the situational awareness to know what’s going on? Now take it a step further: The car sees a moose in the road and says, “I need your help.” Chances are, by the time you put your hands on the wheel and look up, the moose is going to be in your windshield and the situation is going to be in the past. Researchers are looking at how much time it takes to transition back and forth between highly automated or partially automated driving, and fully manual driving. We’ve discovered that it takes a surprising number of seconds for a driver to become engaged after not being focused on the task.
TT: What’s the next new development at SwRI in automated driving research?
SD: We’ve developed more than 15 unique automated vehicles platforms so far. The thing that distinguishes us from the competition is our ability to work in an unstructured environment. Others involved in automated vehicle research have heavily funded their research programs and they do an excellent job of driving down the freeways and down well-marked interior roads. But if only 65 percent of the roadways in this country are paved, how are you going to get a delivery down that last mile on an unpaved road? From a technical perspective, that’s a very different problem. Since 2008 we’ve been working in the military environment. There, we don’t drive on paved roads. We’ve also been successful working for the agricultural equipment and related industries. They’ve got some of the same problems as the military. For example, vineyards aren’t well-defined with pavement between the rows. We don’t have the programs that look for striping on roadways because we’re driving on unstructured environments that have high grass, dirt, and mud. I see us continuing to move some of the capability we developed for the military into the structured environment.
TT: So, what’s next?
SD: Within the industry, across all the companies that are working in the automated vehicle area, we need to integrate ethical algo-rithms into our programs. The reality of automated vehicles is that it’s not just the technology of how do I turn the steering wheel, how do I accelerate, how do I brake. And I think we’ve got to find a way to introduce it to industry and to the public. Frankly, the other thing that’s needed is some legislation and standardization. How will an automated vehicle work in large-scale applications? What are people’s expectations? Like all technologies, these systems are not fail-safe; problems are going to occur. What’s going to happen? Can a company live past that first incident?
Questions about this article? Contact SwRI Solutions.